Lead Follow-Up and Accountability Systems
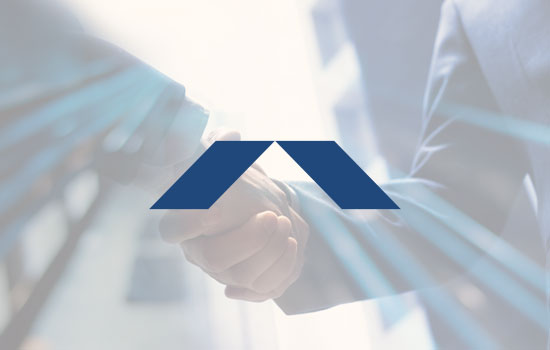
Lead Follow-Up and Accountability Systems: A Scientific Approach
1. The Neuroscience of Follow-Up: Priming and the zeigarnik effect❓❓
Effective lead follow-up leverages cognitive biases to increase conversion rates. Two key principles are:
-
Priming: Introducing a stimulus influences how people respond to subsequent stimuli. In lead generation, initial marketing efforts prime potential clients to be more receptive to follow-up communication. The magnitude of the priming effect, P, can be modeled as:
P = f(S, t, I)
Where:
- S is the strength of the initial stimulus (e.g., marketing campaign).
- t is the time elapsed between the stimulus and follow-up.
- I is the individual’s predisposition or interest in the product/service.
Optimizing S through targeted messaging and minimizing t (while respecting boundaries) maximizes the priming effect.
-
Zeigarnik Effect: People remember incomplete or interrupted tasks better than completed tasks. Initial contact that doesn’t immediately result in a sale creates cognitive tension, making the prospect more likely to remember the agent and respond to follow-up attempts. The probability of recall, R, after interruption can be expressed as:
R = k(1 - C)
Where:
- k is a constant representing the baseline memory capacity.
- C is the completion percentage of the initial interaction.
Thus, lower C (e.g., initial contact without resolution) increases R.
Example: A study by Ferraro et al. (2015) demonstrated that repeated exposure to marketing materials, coupled with strategic follow-up calls, significantly improved brand recall and purchase intent among prospective customers.
Experiment: A/B testing different follow-up schedules based on the initial interaction type can quantify the impact of the Zeigarnik effect on lead conversion. Group A receives follow-up calls 1 day after an open house visit. Group B receives follow-up calls 3 days after the same visit. Conversion rates for each group should be tracked to identify the optimal follow-up timeline.
2. Social Psychology and Lead Conversion: reciprocity❓❓ and Scarcity
Social psychological principles heavily influence lead conversion.
-
Reciprocity: People feel obligated to repay favors or acts of kindness. Providing valuable information or resources upfront without demanding immediate commitment triggers reciprocity. The perceived value of the initial offering, V, directly correlates with the strength of the reciprocal obligation, O:
O = αV
Where:
- α is a constant representing the individual’s sensitivity to reciprocity.
Offering free consultations, market reports, or home valuation estimates can increase V and, consequently, O.
-
Scarcity: Perceived scarcity increases desirability. Highlighting limited-time offers or high demand for properties creates a sense of urgency. The perceived value, PV, of an opportunity under scarcity can be modeled as:
PV = B / S
Where:
- B is the benefit of obtaining the opportunity.
- S is the level of scarcity.
As S decreases (opportunity becomes scarcer), PV increases.
Example: Cialdini’s (2006) work on persuasion extensively explores the power of reciprocity and scarcity in influencing decision-making.
Experiment: Implement two marketing campaigns, both promoting the same property. Campaign A presents the property as widely available, while Campaign B emphasizes its unique features and high demand. Compare the number of inquiries and offers received from each campaign to assess the impact of scarcity on lead generation.
3. Systematized Follow-Up: Markov Chains and State Transition Modeling
A systematized lead follow-up process can be modeled as a Markov chain, where each stage of the follow-up represents a state, and the probability of transitioning from one state to another depends only on the current state.
-
Markov Chain: A sequence of events where the probability of the next event depends only on the present state. In lead management, states can include “Initial Contact,” “Qualified Lead,” “Appointment Scheduled,” “Contract Signed,” and “Closed Deal.”
The transition probabilities are represented by a matrix P, where Pij is the probability of moving from state i to state j.
P = [Pij]
Analyzing the transition probabilities helps identify bottlenecks in the follow-up process and optimize resource allocation.
-
State Transition Diagram: Visual representation of the Markov chain. Nodes represent states, and arrows represent transitions with associated probabilities.
Example: A study by Anderson and Mittal (2000) used Markov chains to model customer behavior in a telecommunications setting, allowing for optimization of customer retention strategies.
Implementation: Use CRM software to track lead progression through different stages. Analyze conversion rates at each stage to identify areas for improvement. For example, if the transition probability from “Appointment Scheduled” to “Contract Signed” is low, investigate the effectiveness of the presentation and negotiation skills of the team.
4. Accountability Systems: game theory❓ and Incentive Structures
Accountability systems should be designed to align individual agent behavior with overall team goals. Game theory provides a framework for understanding and optimizing these systems.
-
Incentive Design: Structuring rewards and penalties to motivate desired behavior. Incentives should be SMART (Specific, Measurable, Achievable, Relevant, Time-bound).
The expected utility, U, of an agent can be expressed as:
U = pB - c
Where:
- p is the probability of achieving the desired outcome.
- B is the benefit or reward associated with the outcome.
- c is the cost or effort required.
To maximize U, the system should increase p and B while minimizing c.
-
Monitoring and Feedback: Regularly tracking and providing feedback on agent performance. This can be achieved through daily/weekly reports, team meetings, and performance reviews.
Example: A study by Lazear (2000) found that performance-based pay significantly increased productivity in a windshield repair company, demonstrating the effectiveness of well-designed incentive systems.
Implementation: Implement a system that tracks key performance indicators (KPIs) like call volume, appointment setting rate, and conversion rate. Provide regular feedback to agents on their performance and offer coaching or training to address areas for improvement. Tie bonuses or other rewards to achieving pre-defined targets.
5. Database Management: Information Theory and Data Optimization
Effective database management is crucial for optimizing lead follow-up. Information theory provides insights into maximizing the value of data.
-
Data Entropy: A measure of uncertainty or randomness in the data. High entropy indicates low information content.
Entropy, H, can be calculated as:
H = - Σ p(xi) log2 p(xi)
Where:
- p(xi) is the probability of occurrence of data point xi.
Reducing data entropy through data cleaning and standardization improves the accuracy and effectiveness of follow-up efforts.
-
Signal-to-Noise Ratio: The ratio of relevant information (signal) to irrelevant or misleading information (noise).
SNR = Psignal / Pnoise
Filtering out irrelevant leads and focusing on qualified prospects improves the SNR, leading to higher conversion rates.
Example: A study by Chen et al. (2004) demonstrated that data quality significantly impacts the effectiveness of data mining and predictive modeling, highlighting the importance of accurate and complete data.
Implementation: Implement data validation rules to ensure data accuracy. Regularly clean the database to remove duplicate or outdated records. Segment leads based on demographic information, interests, and engagement level to target follow-up efforts more effectively.
References:
- Anderson, E. W., & Mittal, V. (2000). Strengthening the satisfaction-profit chain. Journal of Marketing Research, 37(1), 107-120.
- Chen, L., Chiang, R. H., & Storey, V. C. (2004). Business intelligence and analytics: From big data to big impact. MIS Quarterly, 36(4), 1165-1188.
- Cialdini, R. B. (2006). Influence: The psychology of persuasion. HarperCollins.
- Ferraro, R., Shiv, B., & Tanner, R. J. (2015). Priming brand choice: When and why does it work?. Journal of Consumer Research, 42(3), 399-414.
- Lazear, E. P. (2000). Performance pay and productivity. American Economic Review, 90(5), 1346-1361.
ملخص الفصل
Lead follow-up and accountability systems are crucial for optimizing lead conversion❓ rates. The core principle involves assigning responsibility for lead management, defining follow-up timelines, and establishing mechanisms for tracking lead progress. Contact management software serves as the central hub for storing lead data, documenting interactions, and assigning next❓ steps. Effective implementation requires training staff on system utilization, setting performance standards, and holding team❓ members accountable for adherence to protocols. A structured lead management system enhances team efficiency by clarifying individual responsibilities, facilitating performance monitoring, and promoting data-driven decision-making. The key components of a lead follow-up system include: 1. Assignment of responsibility for each lead; 2. Predefined timelines for initial❓ and subsequent follow-up; 3. A centralized database for lead information and interaction history; 4. Documented systems and processes. Successful lead management directly impacts revenue generation by converting potential clients into actual clients. Accountability relies on transparent metrics and regular performance reviews.