Qualifying Leads: Assessing Home Value, Financials, and Expectations
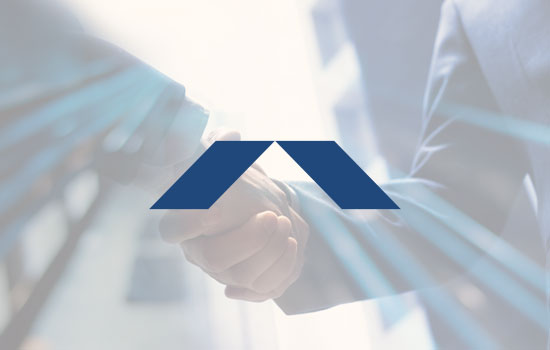
Qualifying Leads: Assessing Home Value, Financials, and Expectations
1.0 Introduction: The Scientific Basis of Lead Qualification
Lead qualification is a critical stage in the sales process, requiring a systematic and data-driven approach. Effectively qualifying leads minimizes wasted resources and maximizes conversion rates. This lesson focuses on three key aspects of lead qualification in real estate: assessing home value, financials, and expectations. These assessments rely on economic principles, statistical analysis, and behavioral psychology.
2.0 Assessing Home Value: Market Analysis and Regression Modeling
The foundation for assessing home value rests on the hedonic pricing model, which decomposes the price of a property into its constituent characteristics. These characteristics include structural attributes (size, age, number of bedrooms/bathrooms), locational attributes (neighborhood quality, school district ratings, proximity to amenities), and environmental attributes (air quality, noise levels).
Equation 1: Hedonic Pricing Model
P = f(S, L, E)
Where:
P = Price of the property
S = Vector of structural attributes
L = Vector of locational attributes
E = Vector of environmental attributes
2.2 multiple regression analysis❓❓:
Multiple regression analysis is employed to estimate the coefficients associated with each attribute in the hedonic pricing model. This statistical technique quantifies the contribution of each characteristic to the overall property value.
Equation 2: Multiple Regression Equation
P = β0 + β1S1 + β2S2 + … + βnSn + βn+1L1 + … + βmEm + ε
Where:
P = Predicted price of the property
β0 = Intercept (base value)
βi = Regression coefficients for each attribute (S, L, E)
Si, Li, Ei = Values of each attribute
ε = Error term
Practical Application: Conducting a Comparative Market Analysis (CMA) involves applying the hedonic pricing model. Agents identify comparable properties (“comps”) that have recently sold and adjust their prices based on differences in key attributes. This requires careful consideration of statistical significance (p-values) associated with each attribute obtained from regression analysis on historical sales data.
2.3 Experiment:
A/B testing different attribute weights within a CMA based on localized regression models. Compare conversion rates and accuracy of pricing estimates with different models.
3.0 Financial Assessment: Risk Analysis and Affordability Metrics
3.1 Debt-to-Income Ratio (DTI):
DTI is a crucial metric for assessing a lead’s financial capacity. It represents the percentage of a borrower’s gross monthly income that goes toward paying debts. A high DTI indicates a greater risk of default.
Equation 3: Debt-to-Income Ratio
DTI = (Total Monthly Debt Payments / Gross Monthly Income) * 100
3.2 Loan-to-Value Ratio (LTV):
LTV compares the amount of the loan to the appraised value of the property. A high LTV indicates a Lower equity stake❓❓ and therefore a higher risk for the lender.
Equation 4: Loan-to-Value Ratio
LTV = (Loan Amount / Appraised Value) * 100
3.3 Net Equity Calculation:
Determining the client’s net equity involves subtracting the outstanding mortgage balance and any liens from the estimated home value. This figure reveals the client’s potential proceeds from the sale.
Equation 5: Net Equity
Net Equity = Estimated Home Value – Outstanding Mortgage Balance – Liens
Practical Application: Accurately estimating the client’s net proceeds allows for realistic expectations and facilitates negotiations. Understanding their DTI and LTV provides insights into their financial flexibility and potential roadblocks in the selling/buying process.
3.4 Experiment:
Simulate different market conditions (interest rate changes, price fluctuations) and assess the impact on clients’ net equity and affordability using Monte Carlo simulations.
4.0 Expectation Management: Behavioral Economics and Cognitive Biases
4.1 Prospect Theory:
Prospect theory, developed by Kahneman and Tversky (1979), explains how individuals make decisions under uncertainty, demonstrating that people weigh potential losses more heavily than equivalent gains.
4.2 Anchoring Bias:
Anchoring bias describes the tendency to rely too heavily on the first piece of information received (the “anchor”) when making decisions. In real estate, this can manifest as an overreliance on initial price estimates.
Loss aversion, a key component of prospect theory, explains why sellers often resist accepting offers below their initial expectations, even if those expectations are unrealistic.
Cognitive dissonance is the mental discomfort experienced when holding conflicting beliefs, values, or attitudes. Sellers may experience dissonance if their expectations are not aligned with market realities.
Practical Application: Understanding these biases enables agents to proactively manage client expectations. This involves providing realistic assessments of home value, explaining market dynamics, and framing offers in a way that minimizes perceived losses.
4.5 Experiment:
Conduct surveys to assess the prevalence of anchoring bias in sellers’ pricing expectations. Compare actual sale prices to initial expectations and analyze the factors contributing to any discrepancies.
5.0 Integrating Assessments: A Weighted Scoring Model
5.1 Model Development:
Develop a weighted scoring model that combines home value assessment, financial assessment, and expectation assessment to generate an overall lead qualification score. Weights are assigned based on the relative importance of each factor in predicting conversion rates and client satisfaction.
Table 1: Example Weighted Scoring Model
Factor | Metric | Weight | Scoring Criteria |
---|---|---|---|
Home Value | Accuracy of CMA | 30% | Deviation from actual sale |
Financials | DTI | 40% | DTI ranges (e.g., <36%, 36-43%, >43%) |
Expectations | Alignment with Market | 30% | Subjective assessment of realism |
5.2 Scoring and Interpretation:
Each lead is scored based on the weighted model. Leads are then categorized into high, medium, and low priority based on their scores. This allows agents to focus their efforts on the most promising leads.
5.3 Iterative Refinement:
The weighted scoring model should be continuously refined based on performance data and feedback. This involves tracking conversion rates, client satisfaction scores, and adjusting the weights and scoring criteria accordingly.
6.0 Recent Research and Studies
- “The Influence of Cognitive Biases on Housing Market Decisions” (2022): A study published in the Journal of Real Estate Finance and Economics examined the impact of anchoring bias and loss aversion on sellers’ pricing decisions. The findings suggest that interventions designed to mitigate these biases can improve negotiation outcomes.
- “Machine Learning Approaches for Automated Property Valuation” (2023): Research presented at the International Conference on Artificial Intelligence explored the use of machine learning algorithms to enhance the accuracy of automated valuation models (AVMs). The study demonstrated that these models can outperform traditional regression-based approaches in certain market conditions.
- “Financial Literacy and Homeownership Sustainability” (2024): A paper in the Journal of Consumer Affairs investigated the relationship between financial literacy, DTI, and LTV, and the likelihood of mortgage default. The results highlighted the importance of financial education in promoting responsible homeownership.
7.0 Conclusion
Qualifying leads effectively requires a scientific approach that integrates market analysis, financial assessment, and behavioral insights. By applying the principles of hedonic pricing, multiple regression, prospect theory, and weighted scoring models, real estate professionals can maximize their efficiency and improve client outcomes. Continuous learning and adaptation based on the latest research are essential for maintaining a competitive edge in the dynamic real estate market.
ملخص الفصل
Home Value Assessment: Subjective homeowner estimations of property value are prone to cognitive❓ biases, leading to inaccuracies. Comparative market analysis❓ (CMA) using recent sales data of similar properties is the scientifically sound method for estimating market value. This mitigates bias by relying on empirical data. Homeowner ratings of property condition on a 1-10 scale, along with identification of desired improvements, allow for objective quantification of perceived value deficits.
Financial Evaluation: Quantitative assessment of outstanding mortgage balances (1st, 2nd, LOC) provides a crucial measure of the homeowner’s financial leverage. Debt-to-equity ratio, calculated from estimated home value and mortgage debt, indicates financial risk. Verifying payment status (up-to-date vs. not) provides evidence of the homeowner’s ability to manage financial obligations. Establishing desired net proceeds from the sale sets a financial benchmark crucial for evaluating the feasibility of a transaction. Determining sole ownership status confirms legal authority to sell, impacting transaction complexity.
Expectation Management: identifying❓ the lead source informs channel-specific conversion rate analysis, a key metric for optimizing marketing resource allocation. Soliciting three specific expectations from a realtor allows for objective comparison with services offered. Understanding whether the lead is interviewing other realtors establishes the competitive landscape and informs sales strategy. Determining the scheduling status of competitor appointments provides a temporal constraint, influencing the urgency and immediacy of follow-up actions.