Lead Management Systems: Tracking and Accountability
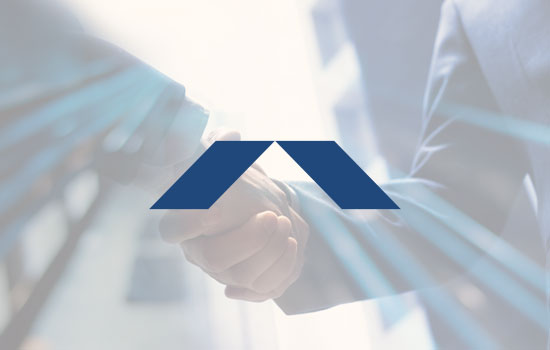
Lead management systems (LMS) are technological applications designed to systematically track, organize, and manage prospective clients, known as leads, throughout the sales process. The scientific importance of LMS lies in their ability to transform unstructured lead data into actionable insights, thereby optimizing resource allocation and conversion rates. Data from behavioral economics suggests that consistent and timely follow-up significantly impacts decision-making, a core principle addressed by LMS functionalities. By providing a centralized repository for lead interactions, LMS facilitate the application of statistical modeling techniques to identify patterns in lead behavior, predict conversion probabilities, and refine marketing strategies based on empirically observed data. The ability of LMS to automatically capture and log interactions ensures compliance with established protocols.
Lead management is a systematic approach to generating, qualifying, nurturing, and converting potential customers into paying clients, integrating marketing and sales efforts. It is underpinned by principles of behavioral economics, data analytics, and marketing automation. Behavioral economics helps understand cognitive biases. Data analytics allows for predictive modeling and targeted interventions through statistical analysis of lead data. Marketing automation uses automated workflows to enhance engagement.
CRM systems are centralized databases for managing customer interactions and lead information. They facilitate data collection, lead segmentation, automated follow-up, and performance reporting. Components include contact management, interaction tracking, lead scoring, and workflow automation.
Lead progression can be modeled using Markov chains, where each state represents a stage in the sales funnel. Transition Probability (Pij) is defined as: Pij = Nij / Ni, where Nij = Number of leads transitioning from state i to state j and Ni = Total number of leads in state i.
Survival analysis❓❓ techniques, such as Kaplan-Meier estimation and Cox proportional hazards regression, can be used to model the time it takes for leads to convert into customers. Hazard Rate (h(t)) is the instantaneous risk of a lead converting at time t. Survival Function (S(t)) is defined as: S(t) = exp(-∫0t h(u) du), where h(u) is the hazard rate at time u.
Data collection strategies include website analytics, form submissions, and social media monitoring.
Accountability metrics are quantifiable measures used to evaluate the performance of individuals and teams involved in lead management processes.
Lead generation metrics include lead volume, lead source effectiveness, and Cost Per Lead (CPL), where CPL = Total Marketing Spend / Number of Leads Generated.
Lead conversion metrics include lead-to-opportunity conversion rate, opportunity-to-customer conversion rate, and Customer Acquisition Cost (CAC), where CAC = Total Sales and Marketing Spend / Number of New Customers Acquired.
Lead nurturing metrics include email open rate, Click-Through Rate (CTR), and lead engagement score.
A/B testing involves comparing two versions of a marketing asset. Leads are randomly assigned to either the control group or the treatment group. Conversion rate is measured for each group.
Multivariate testing involves simultaneously testing multiple variables. Multiple variations of the marketing asset are created. Leads are randomly assigned to each variation and conversion rate is measured.
Predictive lead scoring uses machine learning algorithms to predict the likelihood of a lead converting into a customer.
churn prediction❓❓ involves identifying leads or customers who are at risk of unsubscribing.
Ethical considerations in lead management include data privacy, transparency, and responsible communication.
Chapter Summary
lead❓❓ management systems (LMS) are structured processes leveraging contact management software to optimize lead conversion rates. Effective LMS implementation centers on assigning responsibility, defining timelines, and ensuring data accessibility for each lead.
Key Scientific Points:
- LMS enforce standardized workflows for lead follow-up, minimizing variability and improving predictability of outcomes (aligns with statistical process control).
- Defined roles and responsibilities within the LMS enable clear lines of accountability (leverages the Hawthorne effect).
- LMS facilitate the systematic collection of data on lead sources, conversion rates, and follow-up activities for quantitative analysis❓ to identify bottlenecks, optimize resource allocation, and refine conversion strategies (aligns with evidence-based management).
- LMS provides a framework for training personnel, increasing efficiency and ensuring consistency in lead processing.
- Implementing LMS allows for quantifiable output goals for employees based on conversion data and other system factors.
- LMS promote clarity and consistency in communication, facilitating brand building.
Conclusions and Implications:
Well-designed LMS, coupled with tracking and accountability measures, enhance❓ lead conversion rates, improve operational efficiency, enhance team performance, and increase profitability. The lack of systematic lead management results in wasted resources and missed opportunities including reduced training effectiveness, unclear performance standards, inconsistent branding, and poor resource allocation. Successful LMS deployment necessitates a commitment to data integrity, continuous process improvement, and a culture of accountability.