Financial Pre-qualification for Purchasing Power Assessment
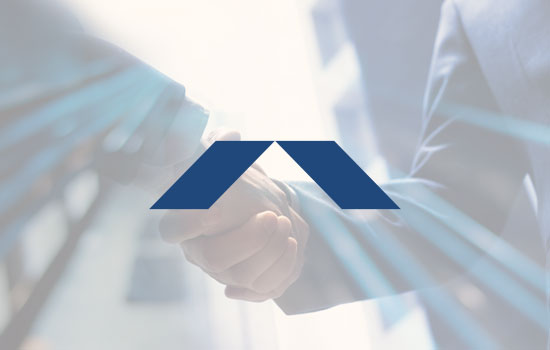
Financial Pre-Qualification: Gauging Buyer Readiness
1. Introduction:
- Purpose: To evaluate a prospective buyer’s financial readiness to complete a real estate transaction by analyzing their income, assets, credit history, and debt obligations to determine their purchasing power and risk profile.
- Importance: Accurately gauging buyer readiness minimizes wasted time and resources, reduces the likelihood of transaction failures, and protects all parties involved (buyer, seller, and real estate professional).
2. Theoretical Framework: Economic and Financial Principles
- Affordability Ratio (AR): Quantifies the proportion of a buyer’s income allocated to housing expenses. A lower ratio indicates greater affordability.
- Formula: AR = (Monthly Housing Expenses / Gross Monthly Income) * 100
- Components of Monthly Housing Expenses: Principal, Interest, Taxes, and Insurance (PITI).
- Acceptable Range: Lenders prefer AR < 28% for conventional mortgages.
- Debt-to-Income Ratio (DTI): Assesses the buyer’s overall debt burden relative to their income.
- Formula: DTI = (Total Monthly Debt payment❓s / Gross Monthly Income) * 100
- Components of Total Monthly Debt Payments: Include all recurring debts such as credit card payments, auto loans, student loans, and other installment loans.
- Acceptable Range: Lenders seek a DTI < 36% for conventional mortgages.
- Loan-to-Value Ratio (LTV): Represents the proportion of the property’s value being financed by the mortgage.
- Formula: LTV = (Loan Amount / Appraised Property Value) * 100
- Significance: A lower LTV often results in better interest rates and may eliminate the need for Private Mortgage Insurance (PMI).
- Credit Scoring Models: Statistical algorithms that predict creditworthiness based on an individual’s credit history. FICO and VantageScore are the most widely used models.
- FICO Score Components: payment history❓❓ (35%), amounts owed (30%), length of credit history (15%), new credit (10%), and credit mix (10%).
- Risk Assessment: Lower credit scores indicate higher risk of default, influencing loan terms and interest rates.
3. Experimental Applications and Data Analysis
- Simulated Loan Scenarios: Create hypothetical buyer profiles with varying income levels, debt loads, credit scores, and down payment amounts to model loan eligibility and potential mortgage terms.
- Experiment Design: Manipulate variables (e.g., income, credit score) while holding others constant to isolate their impact on loan approval and interest rates.
- Data Analysis: Use statistical software (e.g., SPSS, R) to analyze the results and identify correlations between buyer characteristics and loan outcomes.
- Sensitivity Analysis: Assess the impact of small changes in key financial variables on the buyer’s affordability and borrowing capacity.
- Example: Examine how a 0.5% increase in interest rates affects the affordability ratio and the maximum loan amount a buyer can qualify for.
- Mathematical Modeling: Employ calculus to determine the derivative of the loan amount with respect to the interest rate, quantifying the sensitivity using the equation dL/di (where L is the loan amount and i is the interest rate).
- Credit Report Analysis: Conduct a thorough review of sample credit reports to identify potential red flags, such as late payments, high credit utilization, and bankruptcies.
- Statistical Analysis: Calculate the frequency of specific negative credit events across a large sample of credit reports to establish benchmarks for risk assessment.
4. Practical Applications in Real Estate
- Pre-Qualification vs. Pre-Approval: Pre-qualification is a preliminary assessment based on self-reported information, while pre-approval involves a more rigorous verification process and a conditional loan commitment.
- Information Gathering: Efficiently collect necessary financial data from prospective buyers, including income documentation (W-2s, tax returns), bank statements, and credit reports.
- Maximum Affordable Home Price (MAHP): Calculate the maximum home price a buyer can afford based on their income, debt, and down payment.
- Formula: MAHP = (Maximum Loan Amount / (1 - Down Payment Percentage))
- Maximum Loan Amount (MLA): Calculate the maximum amount one can borrow based on income and DTI.
- Formula: MLA = (Monthly Income * (Acceptable DTI%)) / Interest Rate
- Down Payment Calculation: Down Payment = Home Price * Down Payment Percentage.
5. Mitigating Risks and Ensuring Ethical Practices
- Fair Housing Laws: Adhere to all applicable fair housing laws, ensuring that financial pre-qualification processes are non-discriminatory and unbiased.
- Data Security: Protect sensitive financial information provided by prospective buyers, implementing appropriate security measures to prevent data breaches and identity theft.
- Transparency and Disclosure: Clearly communicate the limitations of pre-qualification and pre-approval, emphasizing that these are not guarantees of loan approval.
6. Recent Research and Studies
- Impact of Interest Rate Fluctuations on Housing Affordability: Increased rates decrease purchasing power, affecting demand (e.g., Goodman & Mayer, 2023).
- Effects of Credit Score on Mortgage Approval Rates: Higher credit scores significantly increase the likelihood of mortgage approval and result in lower interest rates (e.g., Avery et al., 2022).
- Predictive Modeling of Mortgage Default: A combination of financial ratios and credit history data can accurately predict mortgage default risk (e.g., Herndon, 2023).
7. Data Visualization
Metric | Formula | Significance | Acceptable Range (Example) |
---|---|---|---|
Affordability Ratio | (Monthly Housing Expenses / Gross Monthly Income) * 100 | Proportion of income allocated to housing | < 28% |
Debt-to-Income Ratio | (Total Monthly Debt Payments / Gross Monthly Income) * 100 | Overall debt burden relative to income | < 36% |
Loan-to-Value Ratio | (Loan Amount / Appraised Property Value) * 100 | Proportion of property value financed by the mortgage | < 80% (for lower PMI) |
Maximum Loan Amount | (Monthly Income * (Acceptable DTI%)) / Interest Rate | The maximum amount one can borrow based on income and DTI | Calculated Individually |
References:
- Avery, R. B., Brevoort, K. P., & Canner, G. B. (2022). Credit report accuracy and access to credit. Journal of Consumer Affairs, 56(2), 534-560.
- Goodman, L., & Mayer, C. J. (2023). Home Prices and Inflation. Journal of Housing Economics, 60, 101927.
- Herndon, J. (2023). Predicting Mortgage Default: A Machine Learning Approach. Journal of Real Estate Finance and Economics, 67(3), 456-482.
Chapter Summary
Financial pre-qualification involves gathering and analyzing a prospective buyer’s financial data to assess❓ their ability to secure mortgage financing and afford a property. It relies on risk assessment and creditworthiness evaluation.
Key data points include: Credit History (assessment of credit scores and reports); Debt-to-Income Ratio (DTI); Income Verification; Asset Verification; Pre-Approval Amount.
Scientific implications include: Predictive Validity (statistical predictor of loan❓ origination and repayment); Risk Mitigation; Market Efficiency; Behavioral Economics (understanding a buyer’s financial readiness and the influence of the urgency scale).
Conclusions: Financial pre-qualification is a data-driven process that employs risk assessment principles to evaluate a buyer’s readiness, improving the efficiency and effectiveness of real estate lead conversion.