Data Collection and Analysis in the Digital Age
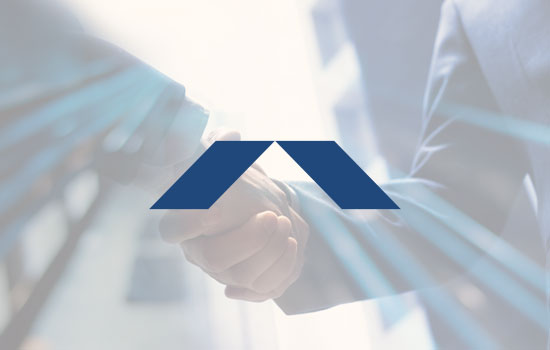
Chapter: Data Collection and Analysis in the Digital Age
Introduction
The digital age has revolutionized real estate❓ appraisal, particularly in data collection and analysis. Traditional methods, while still relevant, are increasingly supplemented and enhanced by digital tools and techniques. This chapter explores these advancements, providing a scientific understanding of the underlying principles and practical applications within the appraisal process.
1. The Foundation: Data and its Significance
At the core of any sound appraisal lies reliable and comprehensive data. Data informs every stage of the valuation process, from defining the problem to reconciling value indicators. The digital age provides access to unprecedented volumes of data, necessitating careful selection, verification, and analysis.
-
Data Types:
- General Data: Macroeconomic indicators, regional and community trends (e.g., employment rates, population growth, interest rates, construction costs).
- Specific Data: Property-specific information like size, features, condition, sales history, and comparable sales data. As mentioned in the provided glossary. “General Data - Data as to real estate values in general. Specific Data - Data pertaining to a particular property.”
-
Data Sources:
- Public Records: County assessor offices, online databases, geographic information systems (GIS).
- Multiple Listing Services (MLS): Comprehensive databases of property listings, sales data, and market trends.
- Commercial Data Providers: Companies like CoreLogic and FNC, Inc., offer extensive property data, analytics, and valuation tools.
- Government Agencies: Census Bureau, Bureau of Economic Analysis (BEA), Federal Housing Finance Agency (FHFA) providing demographic and economic data.
- Mobile Apps: Apps have become more prominent in the field, facilitating on-site data capture.
2. Digital Data Collection Techniques
- Geographic Information Systems (GIS):
- GIS integrates spatial data (location) with attribute data (property characteristics).
- It allows for visualizing property data on maps, identifying comparable properties based on proximity, and analyzing neighborhood trends.
- GIS uses spatial statistics and geostatistical methods to analyze spatial patterns and relationships.
- Example: Using GIS to identify properties within a specific radius of the subject property that share similar zoning and land use characteristics.
- Remote Sensing:
- Satellite imagery and aerial photography provide data on land use, property features, and environmental conditions.
- LiDAR (Light Detection and Ranging) technology creates detailed 3D models of properties, aiding in accurate measurement and assessment.
- Application: Using satellite imagery to assess changes in land use patterns over time, impacting property values.
- Web Scraping:
- Automated data extraction from websites, including real estate portals, government databases, and social media platforms.
- This technique requires ethical considerations and adherence to website terms of service.
- Example: Collecting listing prices, property features, and neighborhood information from multiple online sources to create a comprehensive dataset.
- Mobile Technology:
- Tablets and smartphones facilitate on-site data collection with integrated cameras, GPS, and data entry capabilities.
- Mobile apps allow for capturing property photos, taking measurements, and recording observations, improving efficiency and accuracy.
- Example: Using a mobile app to document property condition, capturing photos of deferred maintenance items that contribute to physical depreciation.
3. Data Analysis: From Raw Data to Meaningful Insights
The digital age facilitates sophisticated data analysis techniques that can uncover patterns and insights difficult to discern through traditional methods.
-
3.1. Statistical Analysis:
- Descriptive Statistics: Measures like mean, median, mode, standard deviation, and range provide insights into data distribution and central tendency. (As defined in the glossary).
- Example: Calculating the average sale price of comparable properties to establish a benchmark for the subject property’s value.
- Regression Analysis: A statistical technique used to model the relationship between a dependent variable (e.g., sale price) and one or more independent variables (e.g., property size, location, features).
- Equation: Y = β₀ + β₁X₁ + β₂X₂ + … + ε
- Y = Dependent variable (Sale price)
- Xᵢ = Independent variables (e.g., square footage, number of bedrooms)
- βᵢ = Regression coefficients (representing the impact of each independent variable on the sale price)
- ε = Error term
- Application: Using regression analysis to quantify the impact of specific property features (e.g., a swimming pool, a renovated kitchen) on sale price, enabling more accurate adjustments in the sales comparison approach.
- Equation: Y = β₀ + β₁X₁ + β₂X₂ + … + ε
- Descriptive Statistics: Measures like mean, median, mode, standard deviation, and range provide insights into data distribution and central tendency. (As defined in the glossary).
-
3.2. Spatial Analysis:
- Techniques used to analyze spatial patterns and relationships in geographic data.
- Hotspot Analysis: Identifies clusters of high or low values, revealing areas with above-average or below-average property values.
- Spatial Autocorrelation: Measures the degree to which nearby properties have similar values.
- Moran’s I: A common measure of spatial autocorrelation:
- I = (N/S₀) * [Σᵢ Σⱼ wᵢ,ⱼ (xᵢ - μ)(xⱼ - μ)] / Σᵢ (xᵢ - μ)²
- N = Number of spatial units (e.g., properties)
- wᵢ,ⱼ = Spatial weight between units i and j (e.g., proximity)
- xᵢ = Value of the variable at location i
- μ = Mean of the variable
- S₀ = Σᵢ Σⱼ wᵢ,ⱼ
- Application: Identifying neighborhoods experiencing rapid appreciation or depreciation, informing the appraiser’s assessment of market trends and influencing value adjustments.
- I = (N/S₀) * [Σᵢ Σⱼ wᵢ,ⱼ (xᵢ - μ)(xⱼ - μ)] / Σᵢ (xᵢ - μ)²
-
3.3. Time Series Analysis:
- Analyzing data collected over time to identify trends, seasonality, and cyclical patterns.
- Moving Averages: Smoothing techniques used to identify underlying trends in noisy data.
- ARIMA Models (Autoregressive Integrated Moving Average): Statistical models used for forecasting future values based on historical data.
- Application: Forecasting future property values based on historical sales data and economic indicators, providing insights into market dynamics and informing investment decisions.
- 3.4. Machine Learning:
- Algorithms that learn from data without explicit programming, identifying complex patterns and making predictions.
- Automated Valuation Models (AVMs): Use machine learning algorithms to estimate property values based on large datasets of property characteristics, sales data, and market trends. (As defined in the glossary).
- Example: Training a machine learning model to identify undervalued or overvalued properties based on a combination of property features, location, and market conditions.
4. Challenges and Considerations
- Data Quality:
- ensuring data❓ accuracy, completeness, and consistency is crucial for reliable analysis.
- Data verification techniques include cross-referencing multiple sources, performing quality control checks, and validating data against known benchmarks.
- Data Bias:
- Recognizing and mitigating potential biases in data is essential to avoid skewed results.
- Examples of bias include selection bias (e.g., using only data from specific neighborhoods) and measurement bias (e.g., inaccurate property measurements).
- Privacy and Security:
- Protecting sensitive property data and adhering to privacy regulations is paramount.
- Data encryption, access controls, and compliance with data privacy laws are essential for maintaining data security.
- Ethical Considerations:
- Using digital tools and techniques ethically requires transparency, objectivity, and avoidance of conflicts of interest.
- Appraisers must adhere to the Uniform Standards of Professional appraisal practice❓ (USPAP) and other ethical guidelines, ensuring that their work is impartial and unbiased.
5. Practical Applications and Experiments
- Sales Comparison Approach Enhancement:
- Use regression analysis to refine adjustments for differences in property characteristics.
- Experiment: Collect data on comparable sales in a specific market and use regression analysis to determine the impact of various factors on sale price.
- Compare the adjusted sales prices derived from regression analysis with those obtained through traditional methods.
- Highest and Best Use Analysis Automation:
- Develop a GIS-based model to evaluate the legal permissibility, physical possibility, and economic feasibility of different land uses.
- Experiment: Use GIS to identify potential development sites in a specific area and analyze their suitability for different land uses based on zoning regulations, topography, and market demand.
- Depreciation Estimation Improvement:
- Utilize machine learning to predict property depreciation based on historical data on property condition, age, and maintenance.
- Experiment: Collect data on property depreciation rates in a specific market and train a machine learning model to predict depreciation based on property characteristics.
- Compare the predicted depreciation rates with those estimated using traditional methods.
Conclusion
The digital age offers unprecedented opportunities to enhance the appraisal process through improved data collection and analysis. By embracing digital tools and techniques, appraisers can increase efficiency, accuracy, and objectivity, ultimately providing more reliable and defensible value opinions. However, it is crucial to address the challenges associated with data quality, bias, privacy, and ethics to ensure that digital advancements are used responsibly and effectively in the appraisal profession.
Chapter Summary
Scientific Summary: data❓ Collection and analysis❓ in the Digital Age for real estate❓ Appraisal
This chapter, “Data Collection and Analysis in the Digital Age,” within the “Mastering Real Estate Appraisal in the Digital Age” training course, addresses the critical role of modern data techniques in real estate appraisal. It emphasizes the shift from traditional, manually intensive data gathering to leveraging digital resources for efficient and accurate valuation. The summary of the key scientific points, conclusions, and implications are as follows:
1. Evolution of Data Collection: The chapter highlights the historical reliance on physical inspections, manual record searches, and limited data availability. The digital age introduces a paradigm shift, enabling appraisers to access vast datasets, including property records, market trends, and geographic information, rapidly and remotely.
2. Digital Data Sources and Types: A core focus is on the identification and utilization of various digital data sources relevant to appraisal. These include:
* Online databases: Public records (deeds, taxes), MLS (Multiple Listing Service) data, assessor data, and specialized appraisal data services (e.g., CoreLogic, FNC, Inc).
* Geospatial data: GIS (Geographic Information Systems) for location analysis, flood maps (FEMA), and environmental data.
* Market data: Economic indicators, demographic trends, and construction costs indices.
The chapter differentiates between *general data* (economic, social, political, and environmental factors) and *specific data* (property characteristics, comparable sales). Emphasis is placed on *verifying* the accuracy and reliability of all data sources.
3. Data Analysis Techniques in the Digital Realm: The chapter discusses how digital tools facilitate advanced data analysis for improved appraisal accuracy. Key techniques include:
* Statistical analysis: Descriptive statistics (mean, median, mode, standard deviation) help understand market trends and property characteristics.
* Regression analysis: To statistically model the relationship between property features and values.
* Automated Valuation Models (AVMs): While acknowledging the limitations, the chapter explores the use of AVMs as a preliminary screening tool or for supporting appraisal conclusions.
* Geospatial analysis: Using GIS software to assess location-based influences on value, such as proximity to amenities or disamenities.
* Paired Data Analysis: Utilizing comparable data to isolate the impact of individual property characteristics on value.
* Relative Comparison Analysis: Ranking comparables based on qualitative characteristics.
4. Impact on Appraisal Approaches: The chapter illustrates how digital data and analysis enhance the three traditional appraisal approaches:
* Sales Comparison Approach: Access to larger comparable sales datasets improves the selection and adjustment process. Digital mapping tools enable detailed❓ location analysis.
* Cost Approach: Online cost estimating services (e.g., Marshall & Swift) provide up-to-date construction costs.
* Income Approach: Digital tools simplify the creation of reconstructed operating statements by providing access to market rent and expense data.
5. Compliance and Ethical Considerations: It underscores the importance of adhering❓ to USPAP (Uniform Standards of Professional Appraisal Practice) when using digital data. Appraisers must:
* Maintain competency in using digital tools.
* Clearly disclose data sources and assumptions in appraisal reports.
* Ensure data accuracy and reliability.
* Address potential bias in data and analysis.
* Properly scope work and intended use.
6. The Future of Appraisal: The chapter concludes by speculating on future trends. This includes increased reliance on machine learning, integration of “FinTech” solutions, and the evolving role of appraisers as data analysts and expert consultants. It is implied that mastery of digital data collection and analysis will be crucial for appraisers to remain competitive and provide credible valuation services in the digital age.